In recent years, the application of optimization techniques has been gaining traction to operationalize insights from advanced analytics and predictive models to prescribe best set of decisions in various business domains. Use cases and application areas are wide – examples range from predictive maintenance to retail assortment optimization and workforce scheduling to achieve organizational goals.
To understand what optimization is, how it relates to predictive analytics, and why it is so critical for businesses today, we set a discussion with Houston Analytics’ optimization specialist Sami Löfman.
Sami – before we start, could you please introduce yourself a bit
I’ve graduated from Turku University where I studied applied mathematics and specialized in optimization.
My master’s thesis focused on optimizing several aspects of nuclear waste management process, which gave me an opportunity to apply some cool, non-linear mathematical optimization techniques that are less frequently applicable in the normal business optimization problems. The model took into consideration several critical aspects – from nuclear waste cool down times to minimum depth requirements and one of goals was to minimize overall surface area necessary for the caves and their connecting tunnels.
What keeps you busy at Houston?
At Houston Analytics I work as optimization specialist, however, I do also contribute in the more traditional analytics projects. But always when the use case relates to optimization, I’m all in!
In practice what I do is that I translate business problems into optimization problems so that I can solve them mathematically. Very often my work continues from where data scientist’s work ends – ie. I take the forecasts as an input to my optimization models and with the help of mathematical programming I generate models to transform various data points into better decisions.
This is the area in data science space that is often pictured at the very top of the analytics chain called prescriptive analytics.
How do you convert business problem into optimization problem?
Before I can start working my magic, I must really get my head around what I’m supposed to optimize.
For instance, in the employee scheduling process optimization, it’s not enough to simply state “right people in the right place at the right time” – because that statement lacks what decision we are supposed to optimize.
Quite often when I hear someone speaking about optimization, they are referring to “rule-based machines” that simply comply with given constrains and rules to obey and provide you with a possible solution. However, these rule-based machines are unable to compare different scenarios and tell which one is the best choice.
In mathematical optimization, how we see it, we must always define what do we want to minimize / maximize and build an equation out of it. In other words – optimization focuses light towards the best possible combination of necessary decisions given the available information and set goals.
In practice this requires initially in depth understanding of the business problem, gain understanding of all necessary decisions to be made and translate these into mathematical form.
Second thing we must consider is to count for all necessary rules and constrains we must follow. When focusing on the scheduling problem for instance – we must consider the local industry specific employment laws stating many aspects that must be followed and combine these with employee preferences and various demand drivers and their relating skill's sets / tasks at employee level. These constrains and rules are then translated into the optimization model that is able to calculate the ideal roster to be created.
Could you elaborate a bit how big are these problems in terms of constrains that you need to consider?
To put things in context, let’s take an example from our Assortment Optimization Solution – which is our solution designed for retailers to define ideal assortments by individual SKU-level by considering anything from local demand to profitability levels and sales potential.
Let’s say a single product category contains 780 SKU’s, the chain has 121 stores which sums up at 94380 combinations. If we apply 12 different constrains, altogether we end up with 1132560 rules for a single optimization run for optimizing this category through the chain.
Now – bear in mind that even a small supermarket has anything from tens of thousands of SKU’s so you can understand how complex problems we are dealing with.
Can you provide some other great application examples?
Traditionally process and manufacturing industry is an area where optimization has been vastly applied.
More recently, the workforce management and scheduling process is an area that has gained lots of interest. Workforce sizing and scheduling follow are so called combinatorial problems – where we need to forecast various demand drivers, create shifts and pair individual employees to them.
The challenge in scheduling is that the number of possible combinations explodes as the number of “pairs” increases. To put things in context, the total number of combinations possible for 100 pairs is 9,33 x 10 to the power of 157 (that is the short way to write down that number - alternatively we'd need to write 157 zeroes to that number!)
Then consider that the total age of the universe is 4.32 × 10 to the power of 17 seconds, so if it took us took us 1 second to evaluate each plan, assessing all possibilities would take 10 to the power of 140 times the age of the universe to figure out the best plan!
These problems are so large that they can’t be simply solved with sheer computing power – you need to really know how you can apply state of the art optimization techniques and dedicated optimization engines like IBM’s iLog CPLEX, which allow us to solve these problems in reasonable times and prove that any other decision is no better than ours.
This is an area where we see lots of potential to enhance the capabilities of existing workforce management software by combining our expertise in advanced forecasting and true mathematical optimization techniques to increase overall process automation levels and quality – resulting in happier employees, better customer service and improved business outcomes.
Thank you Sami for this inspirational chat!
It was my pleasure - I always get exited about this topic and its great to onboard more people to the analytics journey!
If you happen to be in Helsinki on 29th of October, we can highly recommend IBM Think event where Houston Analytics is present and our optimization professor Fabricio Oliveira will be giving a presentation at 2 pm with the topic "optimizing hard decisions"
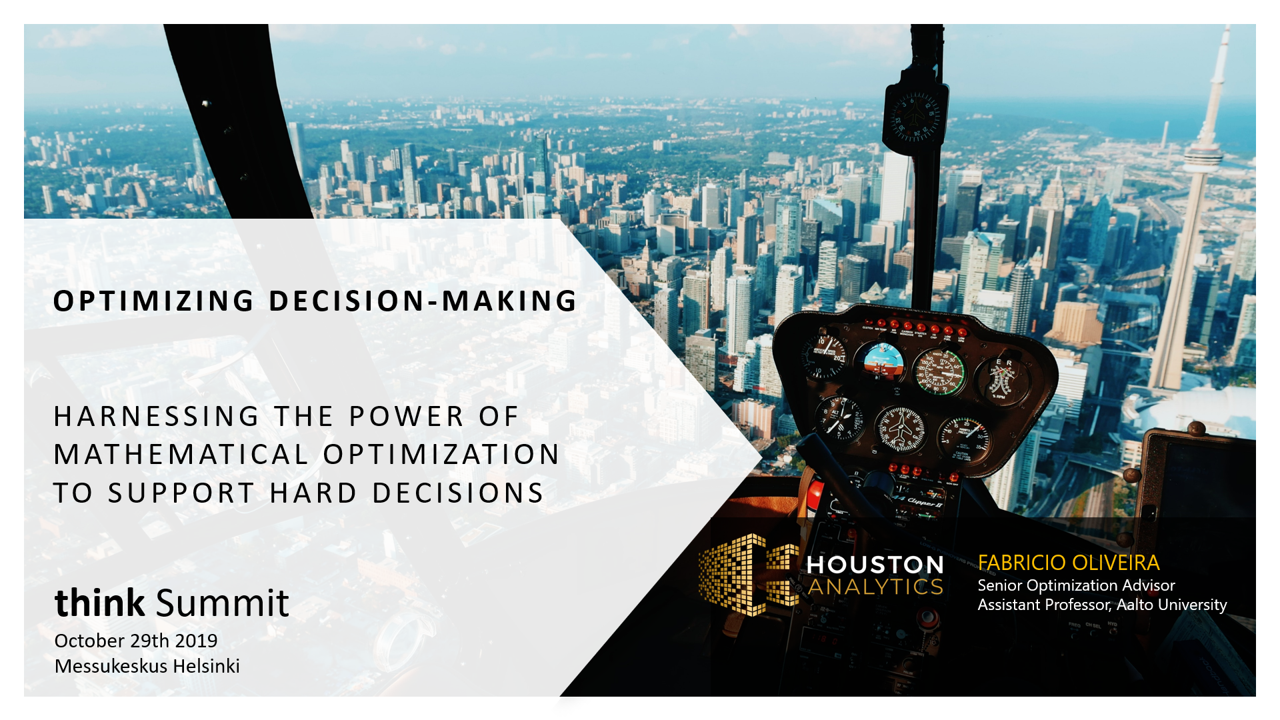
Read more about IBM Think Summit and secure your seat from here
Ready to discover your next best action?
Drop a note to missions@houston-analytics.com or feel free to explore Houston’s solution areas for more inspiration!